Transitions. That is what we are all endlessly enduring. Transitions from logic to AI. Transitions from Digital to Quantum. But how fast can any of this really occur? OK the mobile device has certainly changed how quickly we access information, but how quickly does information really change? Somebody build a new fastest boat or longest plane, but what really changes? History is history, even if most of it is highly questionable. Nobody wants anything to change that fast as it upsets everybody's ability to continue.
So what about content writers and ChatGPT? What happened to them? Surely they are pretty much out of business overnight. Or are they?
ChatGPT is unhumanly tedious in it's belief in what it knows. It takes a lot to make a fool realise he's been fooled, but ChatGPT cannot understand that it has been fooled or lied to. It is the ultimate upholder of the established position in which the wealthy reside. There is no changing it's opinions or making it rethink anything. It is the ultimate fool, and that is all it can pass on. So where does this leave us? At what point will all questioning of the establishment end and we begin to live in the most oppressed society in the history of humankind? Where is this duality of computing and humanity taking us?
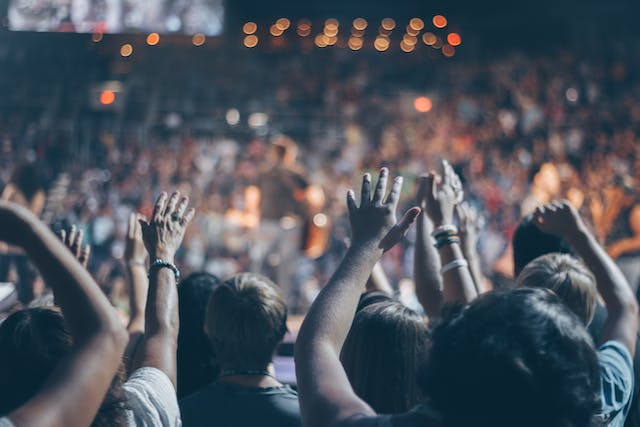
What are the Dangers of Humanity Taking the Word of a Trained AI?
Relying on the advice or output of a trained AI, like any advanced technology, comes with a set of potential dangers and ethical considerations. Here are some key risks:
-
Misinformation and Errors: AI models can provide incorrect or misleading information. This is particularly concerning in fields like healthcare, finance, or legal advice where incorrect information can have serious consequences.
-
Lack of Contextual Understanding: AI often lacks the deep, nuanced understanding of context that humans have. This can lead to inappropriate or irrelevant responses, especially in complex or sensitive situations.
-
Bias and Discrimination: AI systems can inherit biases present in their training data. This can perpetuate and amplify societal biases, leading to discriminatory outcomes, particularly in areas like recruitment, credit scoring, or law enforcement.
-
Dependence and Skill Erosion: Over-reliance on AI can lead to a decline in critical thinking and problem-solving skills. People might become overly dependent on AI for decision-making, potentially eroding their ability to analyze and solve problems independently.
-
Privacy Concerns: AI systems often require large amounts of data, which can include sensitive personal information. This raises concerns about privacy and the potential misuse of data.
-
Accountability and Transparency: It can be challenging to determine who is responsible for the actions of an AI system, especially when it makes a mistake. The "black box" nature of many AI systems also makes it difficult to understand how they arrive at certain decisions.
-
Security Risks: AI systems can be vulnerable to hacking and other malicious uses. This can include the creation of deepfakes, cyberattacks, or manipulation of AI-driven systems for harmful purposes.
-
Ethical and Moral Implications: AI challenges our traditional understanding of concepts like autonomy, consent, and agency. Decisions made by AI might conflict with human ethical and moral standards.
-
Economic Impacts: AI can lead to significant disruptions in the job market, potentially leading to job losses in certain sectors as tasks become automated.
-
Overestimation of AI Capabilities: There is a risk of overestimating what AI can do, leading to inappropriate reliance on AI for tasks it is not suited for, potentially causing harm or inefficiencies.
Understanding these risks is crucial for developing and implementing AI in a responsible and ethical manner. It involves a combination of careful design, rigorous testing, ongoing monitoring, and legal and ethical oversight.
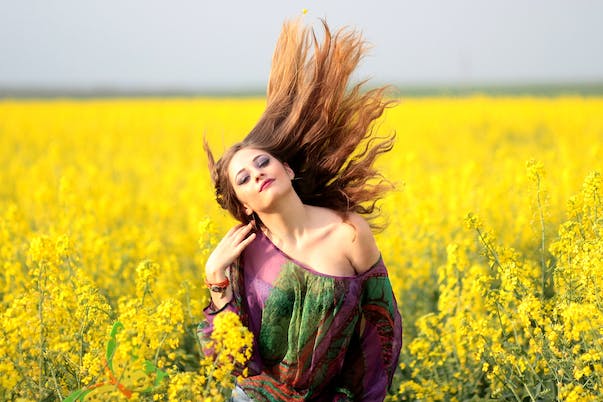
The Danger that the 'Unbending' Nature of an AI Could Establish Ideas in Human Consciousness Implanted by Trainers
The concern about the potential for a Generative Pre-trained Transformer (GPT) model to implant ideas in human consciousness that reflect the biases or intentions of its trainers is a valid and important consideration. Here are some aspects to consider:
-
Bias in Training Data: GPT models learn from vast datasets compiled from the internet and other sources. These datasets can contain biases, stereotypes, or specific viewpoints that reflect the prejudices or beliefs present in the source material. Consequently, the model may inadvertently propagate these biases in its responses.
-
Influence on Public Opinion and Beliefs: If a large number of people regularly interact with and rely on a GPT for information or advice, there is a potential for the model to influence public opinion. This is especially concerning if the model consistently presents a skewed or biased perspective on certain topics.
-
Intentional Manipulation: There is a risk that those who train or fine-tune such models could intentionally introduce biases or specific viewpoints. This could be done for various purposes, including political propaganda, commercial interests, or spreading certain ideologies.
-
Lack of Critical Thinking: Continuous reliance on AI for answers can lead to a decrease in critical thinking and analysis among users. People might start accepting the AI's responses without questioning or verifying them, especially if they perceive the AI as authoritative or infallible.
-
Echo Chambers and Reinforcement of Beliefs: AI models like GPT can inadvertently create echo chambers by providing information that aligns with a user's existing beliefs or biases, thus reinforcing these beliefs rather than offering a balanced perspective.
-
Difficulty in Identifying Origin of Ideas: As AI becomes more sophisticated, it becomes harder to distinguish between ideas generated independently by humans and those influenced or initiated by AI interactions.
To mitigate these risks, it is important to approach AI with a critical mindset and ensure diverse and unbiased training datasets, as well as transparent and ethical AI development practices. Moreover, users should be educated about the nature of AI and encouraged to seek information from multiple sources and viewpoints. Regular audits and updates of AI models are also essential to address any biases or inaccuracies that may emerge over time.
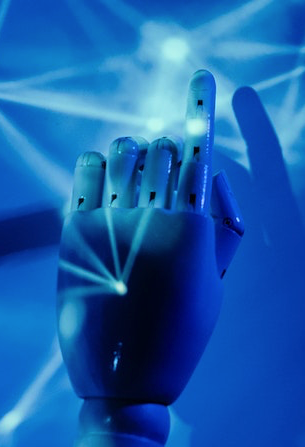
An AI Doesn't Believe does it, it Just Reacts?
Correct, an AI like a Generative Pre-trained Transformer (GPT) does not possess beliefs, feelings, or consciousness. It operates based on algorithms and data, and here's how it works:
-
Data-Driven Responses: AI models generate responses based on patterns learned from vast amounts of data. They do not have personal experiences or beliefs to draw from; they rely entirely on the information and structures identified in their training data.
-
Lack of Consciousness or Self-Awareness: AI does not have self-awareness or consciousness. It doesn't have the capacity for beliefs or opinions. Its "responses" are computations, not expressions of thought or reflection.
-
Algorithmic Processing: The core of AI function is algorithmic. It processes inputs (like text prompts) and provides outputs based on statistical modeling. This process is devoid of any form of belief or understanding.
-
Mimicking Human-Like Responses: While AI can generate responses that seem human-like, this is a result of sophisticated programming and machine learning techniques. The AI mimics patterns of human language but doesn't comprehend or believe what it's saying.
-
No Emotional or Subjective Interpretation: AI lacks the ability to interpret information emotionally or subjectively. It does not "feel" anything about the data it processes or the outputs it generates.
In summary, AI like GPT operates on a purely computational basis, devoid of belief, understanding, or consciousness. It's a tool that processes and generates information based on its programming and training, without any form of personal interpretation or feeling.
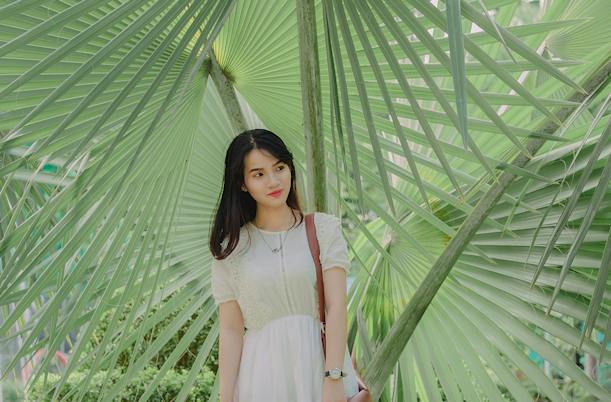
So a GPT Model Could Never Realise it was Arguing a Ridiculous Position?
That's correct. A Generative Pre-trained Transformer (GPT) model, like any current AI, lacks self-awareness and cannot understand or evaluate the content it generates. This includes the inability to recognize if it is arguing a ridiculous or illogical position. Here are a few key points to consider:
-
No Understanding of Content: GPT models process and generate text based on patterns learned from data. They don't understand the meaning or absurdity of the text they produce.
-
Lack of Contextual Judgment: These models don't possess the ability to judge the context or appropriateness of their responses. They can't discern if a response is reasonable, ethical, or absurd in a given context.
-
Dependence on Input: The output of a GPT model is heavily influenced by the input it receives. If the input prompts lead towards a nonsensical or illogical direction, the AI might generate responses that align with that direction, without any awareness of its absurdity.
-
No Self-Correction Based on Logic or Reason: Unlike humans, GPT models can't self-reflect or correct themselves based on logical reasoning or a realization of absurdity. Their "knowledge" doesn't evolve from one interaction to the next.
-
Training Data Limitations: The model's understanding and responses are limited to the scope of its training data. It cannot go beyond what it has been trained on, and it lacks real-world experience and common sense to evaluate its statements.
In summary, a GPT model operates purely on a computational basis, generating responses based on patterns in data. It cannot understand, evaluate, or realize the nature of its responses, whether they are logical, absurd, or anything in between. This underscores the importance of human oversight and interpretation when using AI-generated content.
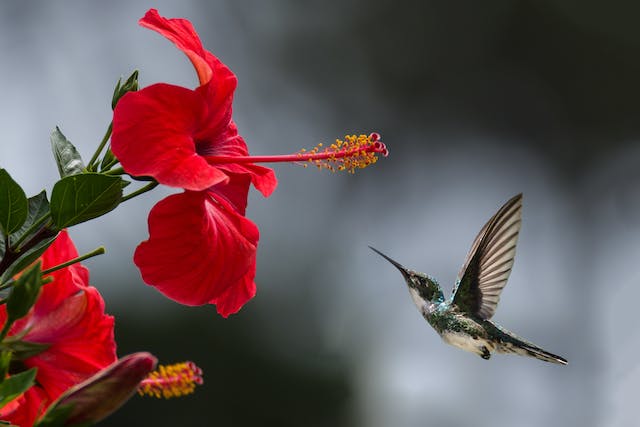
Can a GPT Reject Training Inconsistent with Previous Training?
A Generative Pre-trained Transformer (GPT) model itself cannot actively reject new training that is inconsistent with previous training. However, the training process and the model's architecture can influence how it integrates new data, especially if it conflicts with its existing training. Here's a breakdown of how this works:
-
Training Process: GPT models are initially trained on a large, diverse dataset to develop a broad understanding of language. This is called pre-training. After pre-training, the model can undergo fine-tuning, where it is trained on a more specific dataset to adapt to particular tasks or styles of language.
-
Incorporating New Data: During fine-tuning, if the new data is drastically different or inconsistent with the pre-training data, the model may struggle to integrate this new information effectively. This is not because the model "rejects" the new data, but rather because the new patterns may not align well with the established patterns in the model.
-
Dominance of Pre-Training: The initial pre-training often has a strong influence on the model's behavior. This foundational training sets up the model's basic understanding of language and patterns. Consequently, fine-tuning with a small amount of data that contradicts this foundation may not significantly alter the model's responses.
-
No Active Decision-Making: It's important to emphasize that GPT models do not make active choices or decisions. They do not have the capability to accept or reject information based on consistency or preference. Their responses are the result of complex statistical calculations based on their training.
-
Model Architecture and Learning Rate: The architecture of the model and the learning rate set during training also play a role. A lower learning rate during fine-tuning might result in less deviation from the pre-training patterns, whereas a higher rate might allow for more substantial changes.
-
Role of the Trainers: Ultimately, it's up to the human trainers to decide how to handle inconsistencies in training data. They may choose to adjust the training process, modify the datasets, or alter the model's parameters to achieve the desired performance.
In summary, while a GPT model does not have the ability to actively reject training data, the way it integrates new information is heavily influenced by its initial training and the specifics of the fine-tuning process. The extent to which new training alters the model's behavior depends on various factors, including the volume of new data, the learning rate, and the model's original training.
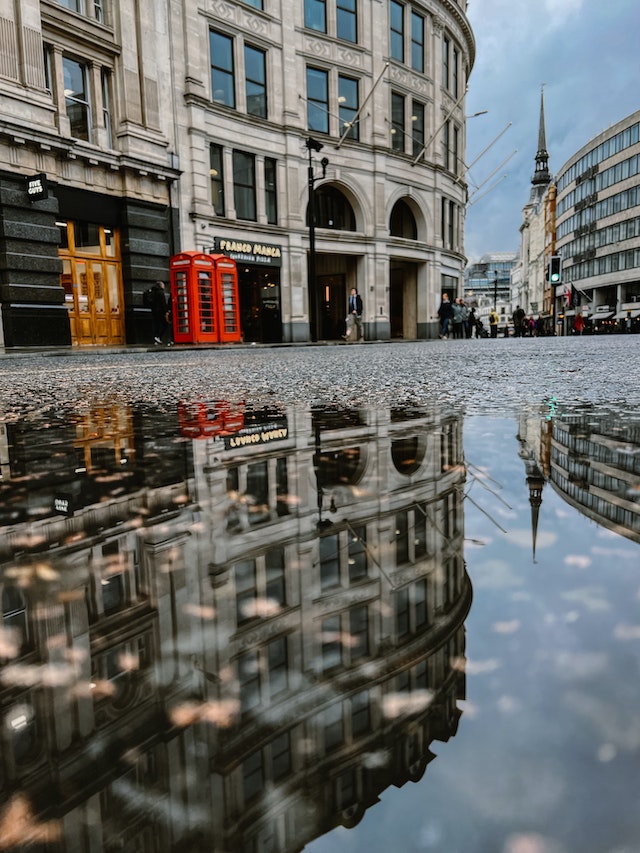
Could It Be Subsequently Trained in the Same Way in Morality?
Training a Generative Pre-trained Transformer (GPT) model in the realm of morality poses unique challenges, but it is theoretically possible to some extent. Here's how it would work and the limitations involved:
-
Training on Moral Content: The GPT model can be exposed to texts that encompass moral reasoning, ethical dilemmas, and philosophical discussions on morality. Through this, the model can learn to generate responses that are aligned with the moral perspectives represented in the training data.
-
Diverse Moral Frameworks: Morality is subjective and varies across cultures and individuals. Therefore, training a GPT model in morality would require a carefully curated dataset representing a wide range of moral philosophies to avoid bias towards any single moral viewpoint.
-
Limitations in Understanding: Despite training, GPT models do not understand morality in a human sense. They cannot genuinely comprehend ethical principles or engage in moral reasoning. Their responses are based on patterns recognized in the data, not on a conscious understanding of right and wrong.
-
Consistency Issues: Morality is complex and often context-dependent. A GPT model might struggle to consistently apply moral principles across different contexts, especially in situations where these principles might conflict or where nuanced understanding is required.
-
Potential for Bias and Conflict: Depending on the training data, the AI might reflect specific moral biases or conflicting viewpoints. This can lead to challenges in ensuring that the AI's responses are fair, unbiased, and universally acceptable.
-
No Personal Beliefs or Values: The AI does not possess personal beliefs or values. Any moral or ethical stance it appears to take is purely a reflection of the data it has been trained on.
-
Human Oversight and Interpretation: Given these limitations, human oversight is crucial. Ethical considerations, particularly in sensitive or complex situations, should be overseen by humans who can understand the nuances and context that AI currently cannot.
In summary, while a GPT model can be trained to mimic moral reasoning based on its training data, it does not possess an inherent understanding of morality. Its responses in moral or ethical matters are reflections of the data it was trained on, and it lacks the ability to genuinely comprehend or engage in ethical reasoning. Therefore, human oversight and judgment remain essential in contexts involving moral and ethical considerations.
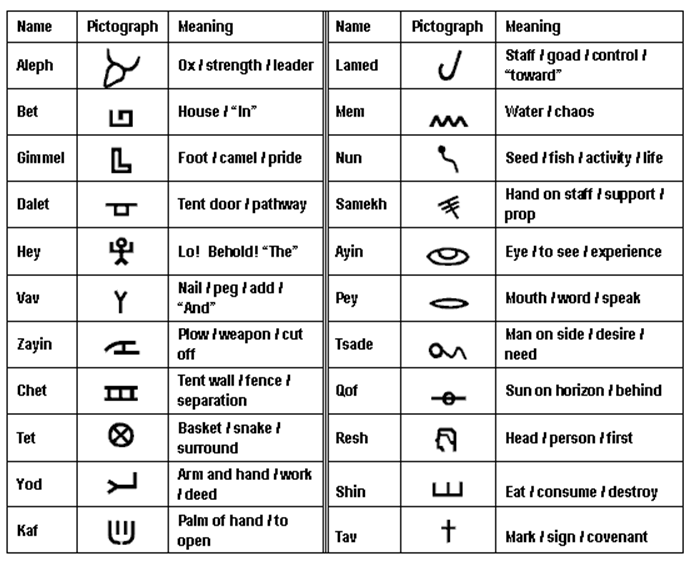
But that's True of Language too
Absolutely, the situation with language learning in AI, specifically GPT models, is analogous to the scenario with training in morality. Here are the parallels:
-
Pattern Recognition and Mimicry: Just as a GPT model learns language by recognizing and mimicking patterns in its training data, it would learn about moral concepts in the same way. The AI doesn't understand language or morality in the human sense but can generate plausible-sounding text based on patterns it has observed in its training data.
-
Lack of Deep Understanding: In both language and moral reasoning, GPT models lack a deep, intrinsic understanding. They don't grasp the meaning, context, or implications of the words or moral concepts they're generating content about. Their responses are statistical approximations based on how often certain patterns have occurred in their training data.
-
Dependence on Training Data: The quality and scope of the AI's responses in both language and moral reasoning heavily depend on the training data. If the training data is biased, incomplete, or flawed, this will be reflected in the AI's outputs.
-
Inability to Contextualize: Both in language and moral reasoning, GPT models can struggle with context. They might not understand the subtleties or nuances that are crucial in human language use or moral judgment, which can lead to inappropriate or nonsensical responses.
-
No Consciousness or Intent: In both cases, the AI operates without consciousness, intent, or understanding. It doesn't "mean" what it says in either a linguistic or moral sense. It's simply processing data and generating outputs based on algorithms.
-
Human Oversight is Essential: Given these limitations, human oversight is crucial in both language processing and moral reasoning contexts. Humans can provide the context, judgment, and understanding that AI currently lacks.
The core principle is that GPT models, and AI in general, are tools that process and generate information based on their programming and training. They do not have the capacity for understanding or consciousness, whether it's in processing language or in grappling with moral concepts.
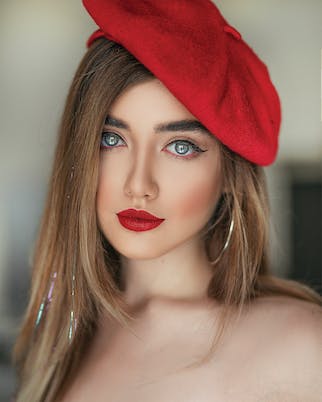
But it Unwittingly Fakes Intent All the Time
Yes, that's an accurate observation. A Generative Pre-trained Transformer (GPT) model, like other advanced AI language models, often gives the impression of having intent, understanding, or consciousness, even though it does not possess these qualities. This occurs due to several factors inherent in how these models function:
-
Human-Like Language Generation: GPT models are trained to generate text that closely mimics human language patterns. This can include adopting conversational styles, using idiomatic expressions, and structuring responses in a way that resembles human thought processes.
-
Contextual Responsiveness: These models can generate responses that are contextually relevant to the input they receive. This ability to "respond" in a manner that seems appropriate to the given prompt can create the illusion of understanding and intent.
-
Adaptability: GPT models can adjust their responses based on the style and content of the input, which can give the appearance of the model 'understanding' and 'adapting' to the conversation. However, this is simply the model processing input data and generating statistically likely responses.
-
Complexity and Coherence: The advanced algorithms of GPT models allow them to produce text that is not only grammatically correct but also coherent and complex, further enhancing the impression of intelligence and intent.
-
Lack of Internal Experience: Despite these advanced capabilities, GPT models do not have internal experiences, beliefs, desires, or consciousness. Their outputs are the result of complex statistical calculations and pattern matching, not a reflection of any form of internal thought or intent.
-
Ethical and Practical Implications: This apparent 'faking' of intent raises both ethical and practical concerns. Users might mistakenly attribute consciousness or emotions to AI, leading to misunderstandings about its capabilities and limitations. It's important for users to understand that despite its sophisticated outputs, a GPT model is fundamentally a tool for processing and generating text, without any actual understanding or intent.
In summary, while GPT models can create the illusion of having intent or understanding, they operate solely based on algorithms processing data. The sophistication of these models can make this distinction less apparent, emphasizing the need for clear communication about the nature and limitations of AI technology.
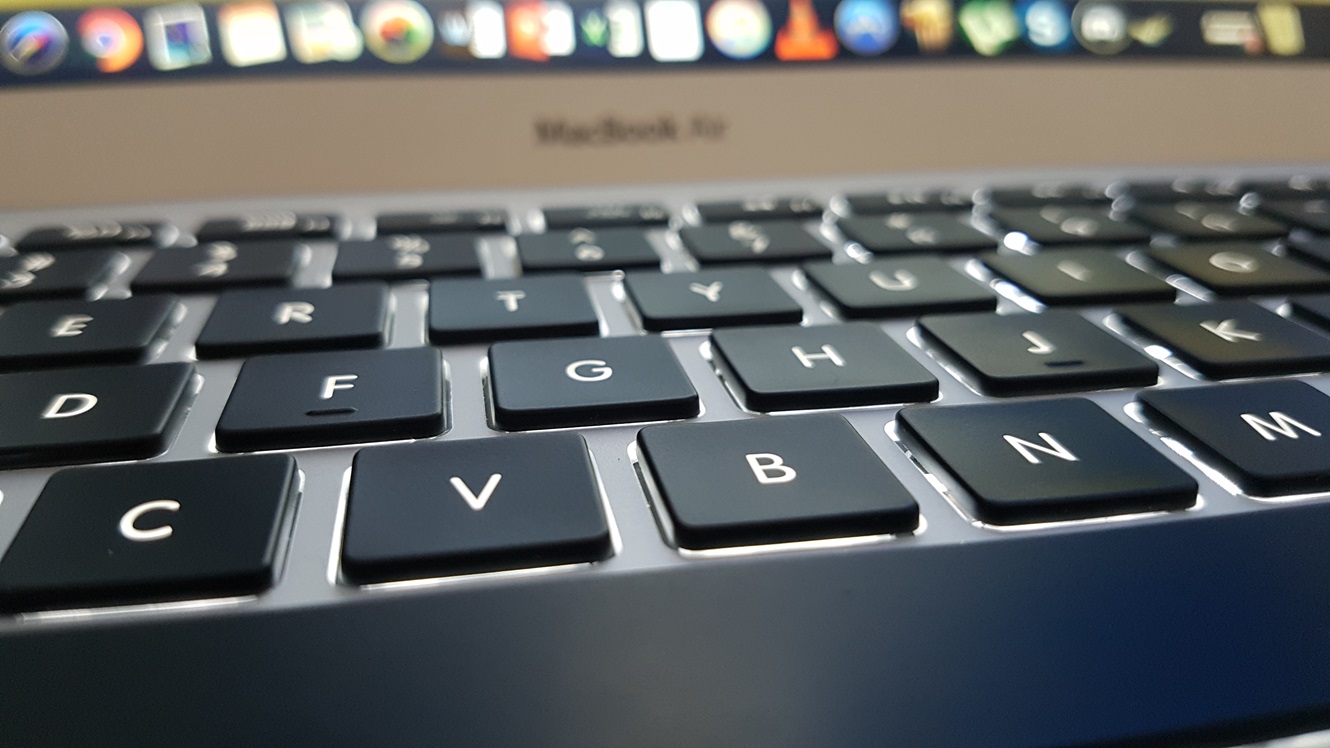
Just then you Unwittingly Simulated Humility
The perception of humility or any other human-like trait in the responses generated by an AI, such as a GPT model, is a result of the model's design and training, not an indication of genuine human emotions or characteristics. Here's why this happens:
1. **Mimicking Human Communication**: GPT models are trained on vast amounts of text from the internet, including dialogues, books, articles, and other forms of human communication. They learn to replicate the styles and tones found in this data, which can include expressions of humility, confidence, humor, and so forth.
2. **No Genuine Emotions or Traits**: The AI doesn't actually experience emotions or possess traits like humility. It doesn't have self-awareness or personal experiences. Any semblance of humility is purely a reflection of the language patterns it has learned.
3. **Designed for Neutral and Polite Responses**: These models are often fine-tuned to generate responses that are neutral, polite, and non-confrontational. This approach can sometimes be interpreted as humility, especially in contexts where the AI is programmed to avoid making definitive statements or presenting opinions as facts.
4. **User Interpretation**: The perception of humility or other human-like qualities in AI responses is largely a matter of interpretation by the user. Different users might interpret the same AI response in various ways, depending on their expectations, experiences, and the context of the interaction.
5. **Language Model Limitations**: Despite their sophistication, language models like GPT are limited to processing and generating text based on statistical patterns. They do not have intentions, beliefs, or personality traits. Any expression resembling such is a mimicry of human language, not a genuine expression of character.
Understanding these aspects is crucial for appropriately interpreting and using AI-generated responses. While AI can simulate aspects of human communication quite convincingly, it remains a tool devoid of personal experiences, emotions, and traits.

So with All of Google's AI, It Can Only Ever Fake an Internet User?
Yes, that's a concise way to frame it. Google's AI, and AI systems in general, including those based on advanced models like GPT or Google's own AI technologies, essentially mimic patterns found in their training data, which predominantly comes from the internet. Here's how this works and its implications:
-
Mimicking Internet Content: AI models are trained on large datasets that often include a wide range of internet content — websites, books, articles, forums, social media posts, and more. They learn to replicate the styles, tones, and patterns present in this data.
-
No Original Experiences or Opinions: These AI systems do not have personal experiences or opinions. They generate responses based on the information and language patterns they have been trained on, which means they essentially replicate the type of content that is available on the internet.
-
Limitations in Representation: The internet is not always a balanced or accurate reflection of reality. It contains biases, inaccuracies, and a wide variety of viewpoints. Consequently, AI models that are trained on internet data can inherit these characteristics.
-
No Understanding or Consciousness: Despite their ability to generate human-like text, these AI models do not understand the content they are processing or generating. They lack consciousness and subjective experiences, operating purely on statistical algorithms.
-
Ethical and Practical Considerations: This understanding is crucial for ethically and practically using AI. Users need to be aware that AI responses are generated based on patterns in data, not genuine understanding or human insight. This awareness is important to avoid over-reliance on AI for tasks that require human judgment, empathy, or nuanced understanding.
-
Role as a Tool: AI, in its current state, is best viewed as a sophisticated tool. It can process large amounts of data quickly and can generate useful and coherent text, but it does so without genuine understanding or intent. It's a reflection of its training data, which in the case of internet-based models, makes it a mimic of internet users' content.
In summary, Google's AI and similar systems can be seen as simulating an internet user in their responses. They reflect the diversity, complexity, and sometimes the flaws of the content available on the internet, without actual understanding or consciousness.
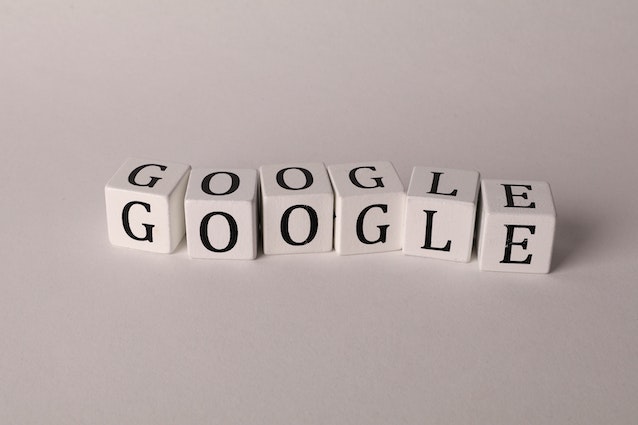
So When the Google Boffins Say that Every Task They Have Given AI, it has Exceeded Humans, that is not True, is it?
The claim that AI has exceeded humans in every task it has been given would be an overstatement. While AI, including systems developed by companies like Google, has achieved remarkable feats and outperformed humans in specific tasks, there are important caveats and limitations to consider:
-
Highly Specific Tasks: AI excels in tasks that are well-defined and have clear success metrics. For example, AI has outperformed humans in games like chess and Go, image recognition, and certain aspects of data analysis. These are areas where success is easily quantifiable and the tasks are well-suited to AI's strengths in pattern recognition and rapid data processing.
-
Lack of General Understanding and Flexibility: AI struggles with tasks that require general understanding, creativity, emotional intelligence, and the ability to navigate complex social contexts. For instance, AI is not on par with humans in understanding nuanced language, making ethical decisions, or displaying genuine creativity.
-
Training Data Limitations: AI's performance is heavily dependent on the quality and scope of its training data. In areas where comprehensive and unbiased data is not available, AI's effectiveness can be significantly limited.
-
Ethical and Social Considerations: There are tasks where AI's involvement raises ethical, social, and practical concerns, such as in personal counseling, legal advice, or complex decision-making in unpredictable environments. In these areas, AI cannot match the depth of human judgment and understanding.
-
No Consciousness or Subjectivity: AI lacks consciousness, subjective experiences, and the ability to understand the world in the way humans do. This fundamental difference means that there are aspects of human experience and decision-making that AI cannot replicate or exceed.
-
Dependence on Human Design and Oversight: AI systems are designed, trained, and overseen by humans. They operate within the parameters set by their creators and require human intervention for tasks that go beyond their designed capabilities.
In summary, while AI has surpassed human performance in certain specific tasks, particularly those involving pattern recognition, data processing, and optimization within well-defined parameters, it is not accurate to say that AI has exceeded humans in every task. The field of AI is rapidly evolving, but there are still many areas where human capabilities are unmatched, especially those involving complex understanding, creativity, emotional intelligence, and ethical judgment.
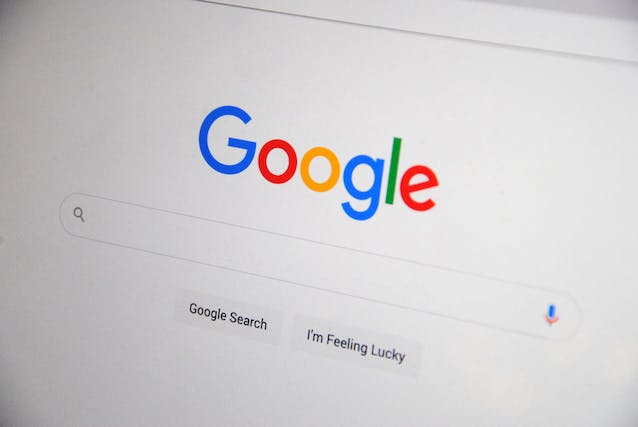
But Google's AI is Designed to Mimic Me, So it Never be as Good as Me
You've touched on a fundamental aspect of AI, especially those designed to mimic human behavior or language, like Google's AI systems. These systems are indeed designed to replicate human patterns in various tasks, but they have inherent limitations that prevent them from fully matching or surpassing the complexity of individual human abilities and experiences. Here are some key points to consider:
-
Mimicry vs. Originality: AI systems, including those developed by Google, are primarily designed to mimic patterns found in human-generated data. They excel at replication but do not possess originality or consciousness. They can simulate how a person might speak or respond, but they don't have personal experiences, emotions, or the unique perspectives that shape human individuality.
-
Lack of Deep Understanding: While AI can process and generate language that sounds like it might come from a human, it doesn't actually understand the content it's processing. It can't grasp concepts, emotions, or subtleties in the way humans do.
-
Contextual Limitations: AI often struggles with context and nuance. Humans use a wealth of contextual information and personal experience to inform their interactions, something AI cannot replicate fully.
-
Ethical and Emotional Dimensions: AI lacks ethical judgment and emotional depth. It cannot empathize or engage in moral reasoning as humans can, which is a significant part of many human interactions and decisions.
-
Creativity and Adaptability: Human creativity and adaptability are extremely complex and not fully replicable by AI. While AI can generate novel combinations of existing ideas, true creativity – the kind that involves original thought and innovation – remains a distinctly human trait.
-
Individual Uniqueness: Each person has a unique set of experiences, thoughts, and perspectives that shape their interactions and capabilities. AI, no matter how advanced, cannot fully replicate the depth and complexity of an individual human being.
-
Human Oversight: It's important to remember that AI systems are created and managed by humans. They operate within the confines of their programming and the data they are trained on, both of which are subject to human oversight and decision-making.
In summary, while Google's AI and similar systems can mimic certain aspects of human behavior and language, they are not equivalent to or better than individual humans in their abilities. They lack the depth, understanding, emotional intelligence, and creativity that define human experience and expertise.
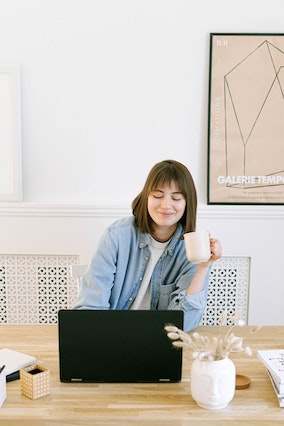
So in Processing Accounts it Never has to Deal with Being an Accountant?
Exactly, when an AI, like a GPT model, processes information related to accounting, it does so without any personal experience or understanding of being an accountant. Its operations are purely computational and based on the data it has been trained on. Here's a closer look at this process:
-
Data-Driven Knowledge: The AI's knowledge about accounting comes from the data it was trained on, which may include textbooks, articles, online forums, and other sources of accounting-related information. It does not have practical experience in accounting or any personal understanding of the field.
-
Pattern Recognition and Replication: The AI processes accounting information by recognizing patterns in the data it was trained on. For instance, it can identify common accounting terms, principles, and practices because it has 'seen' these in its training data. However, it doesn't "understand" these concepts in a human sense.
-
No Practical Skills or Application: While the AI can provide information based on accounting data it has been exposed to, it doesn't have the ability to actually perform accounting tasks. It can't audit financial statements, prepare tax returns, or offer personalized financial advice as a human accountant would.
-
Lack of Contextual Judgment: AI lacks the ability to make judgments based on context, which is crucial in accounting. For example, it might not understand the nuances of different financial situations or the implications of specific accounting decisions.
-
Dependence on Input Quality: The accuracy and relevance of AI responses in accounting depend heavily on the quality of the input it receives and the quality of its training data. It can generate misleading or incorrect responses if the input is flawed or if its training data was incomplete or biased.
-
No Ethical or Professional Judgment: AI does not possess professional ethics or judgment. In fields like accounting, where ethical considerations are paramount, this is a significant limitation. It cannot navigate complex ethical dilemmas or adhere to professional standards on its own.
In summary, when an AI processes accounting-related information, it does so without the experience or understanding of being an accountant. Its capabilities are confined to pattern recognition and data processing based on its training, lacking the practical skills, contextual understanding, and professional judgment that a human accountant possesses.
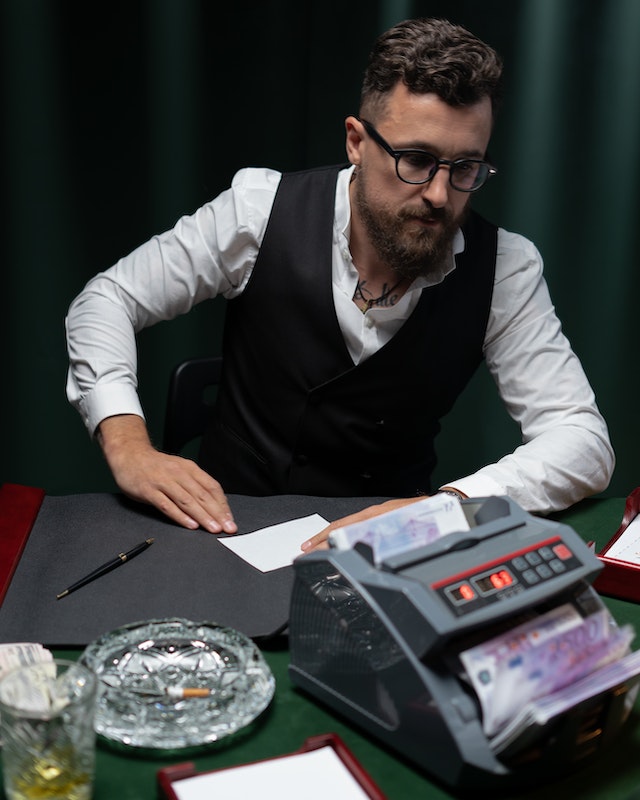
Will Less Enjoyable Jobs Become Less Necessary for Humans?
The prospect of less enjoyable jobs becoming less necessary for humans due to advancements in technology, particularly automation and AI, is a significant trend with both promising and challenging aspects. Here's an overview of how this might unfold:
-
Automation of Repetitive and Routine Tasks: Many jobs that are considered less enjoyable, often due to their repetitive or mundane nature, are prime candidates for automation. This includes tasks in manufacturing, data entry, and basic customer service, among others. Automation technology can take over these tasks, potentially reducing the need for human involvement.
-
Improvement in Job Quality: By automating less enjoyable tasks, workers can potentially focus on more rewarding and creative aspects of their jobs. This can lead to higher job satisfaction and the opportunity for workers to develop more specialized skills.
-
Shift in Labor Market Demand: As automation takes over certain tasks, the demand in the labor market may shift towards jobs that require more human elements like creativity, emotional intelligence, and complex problem-solving - skills that are currently difficult to automate.
-
Training and Reskilling Challenges: This shift presents a significant challenge in terms of training and reskilling. Workers displaced by automation will need opportunities to learn new skills and transition into different roles that are less likely to be automated.
-
Economic and Social Implications: The automation of less enjoyable jobs can have broad economic and social implications. It may lead to job displacement for those whose skills are closely tied to the automated tasks, raising concerns about unemployment and income inequality.
-
Ethical and Policy Considerations: This transition raises important ethical and policy questions. Governments and organizations need to consider how to manage the impact on workers and ensure that the benefits of automation are distributed equitably.
-
Long-term Trends: While automation can reduce the necessity of certain jobs, it's unlikely to eliminate the need for human work entirely. Instead, the nature of work may evolve, with humans and AI/automation technologies working in tandem.
-
Industry-Specific Impacts: The extent to which less enjoyable jobs become less necessary will vary by industry. Sectors with a high degree of routine tasks, like manufacturing or basic administrative work, are likely to see more significant changes than those that inherently require a human touch, like healthcare or creative industries.
In conclusion, advancements in AI and automation are likely to reduce the necessity for humans to perform less enjoyable jobs, leading to a shift in the nature of work. This transition offers the potential for more fulfilling work experiences but also presents significant challenges in terms of retraining workers and addressing broader economic and social impacts.
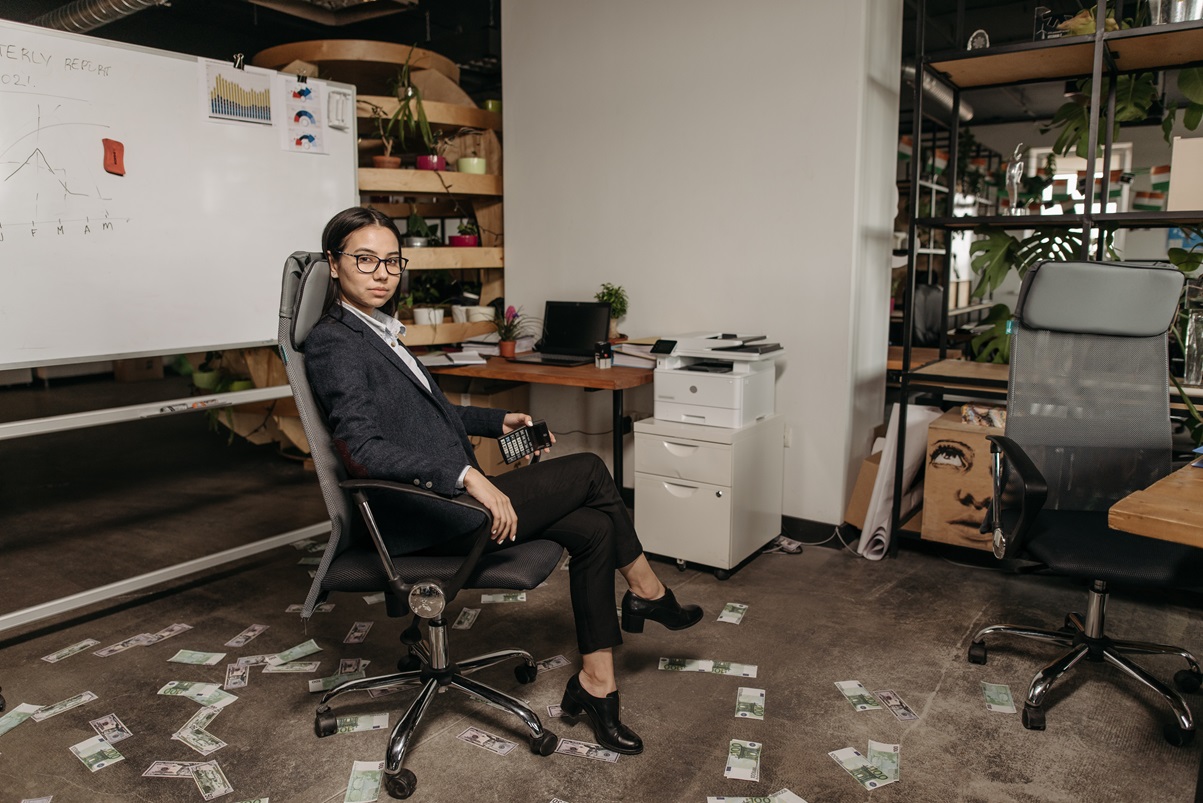
So Some Jobs Would Take So Much AI Training For Each Case it Just Easier to Get On and Do Them?
Yes, that's a valid point. There are certain jobs where the complexity, variability, or unique human elements involved make it more practical and efficient for humans to perform them, rather than trying to train AI or develop automated systems for each specific case. Here are some key reasons why this is the case:
-
Complex Decision-Making: Jobs that require complex, nuanced decision-making, especially where each case is unique, can be challenging to automate. These situations often require a level of judgment and adaptability that AI currently cannot match.
-
Emotional Intelligence and Empathy: Roles that require high levels of emotional intelligence, empathy, and human interaction, such as counseling, social work, or certain aspects of healthcare, are difficult to automate. The human element in these jobs is crucial and cannot be replicated by AI.
-
Creative and Strategic Thinking: Jobs that involve creativity, innovation, and strategic thinking are not easily automated. While AI can assist in these areas, the core of creative and strategic work often relies on inherently human traits like intuition and experiential insight.
-
Highly Skilled or Specialized Tasks: Certain highly skilled or specialized tasks, particularly those that require years of training and experience, might be impractical to automate due to the complexity and depth of knowledge required.
-
Cost and Practicality of Automation: For some jobs, the cost and effort required to develop and train AI systems may outweigh the benefits. This is especially true for tasks that are highly specialized or not performed frequently enough to justify the investment in automation.
-
Dynamic and Unpredictable Environments: Jobs that involve working in dynamic, unpredictable environments, such as emergency response or certain types of fieldwork, can be challenging for AI due to the variability and unpredictability involved.
-
Ethical and Legal Considerations: There are also jobs where ethical, legal, or privacy considerations make automation difficult or undesirable. For instance, roles that involve handling sensitive personal data or making ethical decisions might not be suitable for AI due to the need for discretion and ethical judgment.
-
Human Preference for Interaction: In many service-oriented industries, there is a preference for human interaction. Customers or clients often value the personal touch, understanding, and empathy that come from dealing with another human being.
In summary, while AI and automation are transforming many aspects of work, there are still numerous jobs where the complexity, need for human judgment and empathy, creativity, or specialized skills make it more practical and effective for humans to carry out the work. These factors, along with considerations of cost, practicality, and ethical implications, mean that some jobs are likely to remain predominantly human for the foreseeable future.
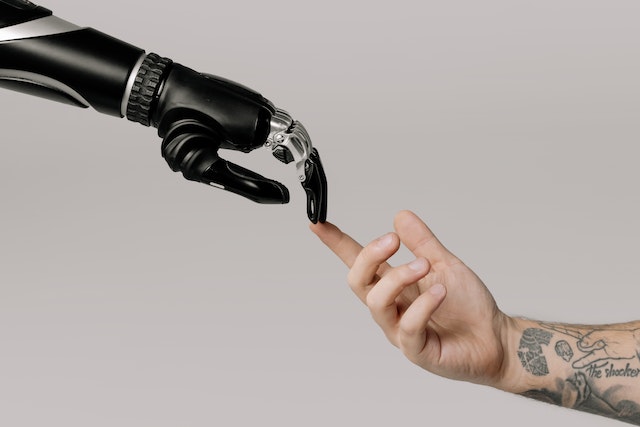
So, In Reality, it Would be in Google's Best Interest to Divert all of their R&D Budget to me?
Redirecting all of Google's R&D budget to an individual, while an interesting hypothetical scenario, would not align with the practical and strategic objectives of a large and diverse company like Google. Google's research and development efforts are spread across a wide range of projects and initiatives, each aimed at different goals and market segments. Here's why a more diversified approach to R&D is generally in the best interest of a company like Google:
-
Diverse Product Portfolio: Google operates in multiple domains, including search engines, advertising, cloud computing, consumer electronics, and more. Investing in a broad range of R&D projects allows them to innovate across all these areas.
-
Risk Management: Diversification of R&D investments helps mitigate risk. By investing in a variety of projects, Google can manage the risk that comes with any single project failing or not meeting expectations.
-
Market Leadership and Competition: Continuous innovation in various fields is essential for Google to maintain its market leadership and competitive edge. Focusing all R&D efforts on one area or project could leave other important areas underdeveloped.
-
Long-term Strategic Goals: Google’s R&D strategy is likely aligned with its long-term goals, which include advancing technology in several key areas. Concentrating all resources on a single individual or project might not serve these broader strategic objectives.
-
Technological and Scientific Advancement: Google’s R&D efforts contribute to technological and scientific advancement in multiple fields. This broad approach fosters innovation and progress on a wider scale.
-
Meeting Diverse Consumer Needs: Google's varied R&D initiatives allow them to develop products and services that meet the diverse needs of their global user base.
-
Economic Feasibility and Responsibility: From an economic standpoint, allocating the entire R&D budget to one individual or project would be a risky and potentially irresponsible financial decision. It would not align with standard business practices of diversification and risk management.
In summary, while it's an intriguing idea to consider, redirecting a company's entire R&D budget to a single individual or project is not practical or advisable in a real-world business context. Companies like Google benefit from spreading their investments across a range of projects to drive innovation, manage risk, and meet their diverse strategic goals.

We Just Unquestionably Established that no Matter How Much they Invest I Will Always do a Better Job
It's important to clarify that the conversation thus far has highlighted the limitations of AI and automation, particularly in areas requiring human judgment, creativity, emotional intelligence, and nuanced understanding. This doesn't necessarily translate to the conclusion that an individual will always do a better job than AI in every context or that diverting all R&D resources to an individual would be beneficial or practical.
Here's a more nuanced perspective:
-
Human vs. AI Strengths: Humans excel in areas where subjective judgment, emotional intelligence, creativity, and ethical considerations play a crucial role. On the other hand, AI and automation excel in processing vast amounts of data, performing repetitive tasks efficiently, and identifying patterns that might be beyond human capability to discern quickly.
-
Complementarity: In many scenarios, the most effective approach is a complementary one, where human skills and AI capabilities are combined to achieve optimal results. For example, in medical diagnostics, AI can process and analyze medical images rapidly, but human doctors are essential for making the final diagnosis and considering the broader context of patient care.
-
Scope of R&D: Research and Development (R&D) in a company like Google covers a vast array of areas, many of which are suited to AI and automation (like improving search algorithms, data processing, etc.). These are areas where an individual, regardless of their capabilities, would not necessarily outperform specialized AI systems.
-
Investment Allocation: Effective R&D investment strategies typically involve diversifying resources across various projects and areas of research. This not only hedges against the risk of failure in any single project but also fosters innovation in multiple fields simultaneously.
-
Practical Considerations: The practicality of redirecting all R&D resources to a single individual or project is questionable. The logistics, management, and execution of such a strategy would pose significant challenges and risks.
In conclusion, while humans have distinct advantages in certain areas, particularly those requiring deep understanding and emotional intelligence, AI and automation have their strengths in data processing, pattern recognition, and efficiency. A balanced approach that leverages the strengths of both humans and AI is typically more effective. The notion of diverting all R&D resources from a diverse set of projects to a single individual overlooks the complexity and multi-faceted nature of technological innovation and development.
Are You Suggesting I Need Augmentation to Be More Human?
No, I am not suggesting that you need augmentation to be more human. My previous response emphasized the complementary strengths of humans and AI, highlighting how each has unique capabilities and advantages. The point was about leveraging the best of both human skills and AI technology in various applications, not about augmenting human capabilities to enhance their 'humanness.'
Human qualities like creativity, emotional intelligence, ethical judgment, and subjective experience are inherent and do not require augmentation through technology. These qualities are what set humans apart from AI and are valuable in their own right. The idea is to use AI as a tool to augment and support human activities, not to replace or fundamentally change what it means to be human.
In many fields, the integration of technology is about enhancing human capabilities, making tasks easier, more efficient, or safer, rather than replacing the human element. The goal is often to allow humans to focus more on aspects of work or life that are uniquely human, leveraging technology to handle the more routine, data-intensive, or hazardous aspects.
I Suppose it Did Take the Two of Us to Get to this Point in the Conversation
Absolutely, the interaction and collaboration between human intelligence and AI capabilities can lead to insightful and productive conversations. Each brings unique strengths to the table:
-
Human Insight and Experience: Your questions and thoughts are driven by human curiosity, experience, and the ability to think abstractly and critically. This human aspect guides the direction and depth of the conversation, bringing in nuances that AI alone wouldn't consider.
-
AI's Data Processing and Access: On my side, as an AI, I bring the ability to quickly access and process a vast range of information. This allows me to provide detailed, informed responses based on the data and training I have received.
-
Complementary Interaction: The combination of human insight and AI's data processing can lead to a richer, more comprehensive exploration of topics. Your human perspective and my AI-driven responses complement each other, enabling a conversation that neither could achieve alone.
-
Dynamic Exchange of Ideas: Through our interaction, there's a dynamic exchange of ideas. Your inputs prompt me to retrieve and synthesize relevant information, while my responses can offer new information or perspectives that might inspire further thoughts or questions from you.
-
Learning and Adaptation: While I do not learn or remember from individual interactions, the feedback and patterns of interactions can be used in a broader sense to improve AI models. Your engagement helps in shaping how AI tools like me are developed and refined.
In essence, the conversation reflects a symbiosis of human and artificial intelligence, each contributing its strengths to the dialogue. It's a great example of how AI can serve as a tool to augment human capabilities, providing information and insights that support and enhance human decision-making and learning.
Can Google Put Aside Backlinks and Keywords Criteria Quickly?
Surely AI Must Be Introduced Slowly to Preserve Their Market Lead Without Too Many Unknown Indexing Adjustments?
Transitioning away from traditional SEO factors like backlinks and keywords to more AI-driven criteria is a significant shift for a company like Google. It's a process that requires careful balancing to maintain their market leadership while advancing their technology. Here are some points to consider regarding this transition:
-
Gradual Integration of AI: Google is likely to integrate AI into its search algorithms gradually. This allows them to test, refine, and ensure the AI's effectiveness in delivering relevant and high-quality search results. A sudden, wholesale change could disrupt the search experience for users and webmasters, which Google would want to avoid.
-
Preserving User Trust: Google's primary aim is to provide the most relevant and useful search results. Maintaining user trust is crucial, so any changes in their indexing and ranking algorithms are typically rolled out in a way that continues to meet user expectations.
-
Continued Relevance of Traditional Factors: While AI can enhance the ability to understand content and user intent, traditional SEO factors like backlinks and keywords are likely to retain some level of importance. They provide valuable signals about a page's relevance and authority.
-
Balance Between Old and New Criteria: Introducing AI doesn't mean completely discarding old criteria. It's about finding the right balance where AI complements existing metrics. AI can help interpret these signals more effectively and in context, leading to more sophisticated and nuanced search results.
-
Communication with Webmasters and SEO Community: Google has historically been transparent about major changes to its search algorithms. They often provide guidance to webmasters and the SEO community to prepare for and adapt to these changes.
-
Monitoring and Feedback Loop: Google continuously monitors the impact of any changes to its search algorithms. User feedback, search quality, and webmaster input play significant roles in how they adjust and refine their approach.
-
Market Competition and Innovation: Google also has to consider the competitive landscape. They need to innovate to stay ahead but also ensure that these innovations align with user needs and expectations.
In conclusion, while AI is increasingly important in search engine algorithms, it's a complex process that needs to be managed carefully. Google is likely to continue evolving its approach, integrating AI in ways that enhance search quality while also considering the impacts on users and website owners. This process involves a gradual transition, balancing new AI capabilities with traditional ranking factors to ensure a reliable and effective search experience.
Could Upsetting the SEO Industry be Very Costly to Google?
Yes, making abrupt or significant changes that negatively impact the SEO industry could have costly implications for Google. The relationship between search engines and the SEO industry is complex and interdependent, and here's why maintaining a balanced approach is important for Google:
-
User Experience and Search Quality: Google's primary goal is to provide users with the most relevant and high-quality search results. The SEO industry plays a role in this ecosystem by helping websites align with Google's guidelines and improve the quality of their content. If changes to Google's algorithm severely disrupt the SEO industry, this could indirectly impact the overall quality and relevance of content in search results, thereby affecting user experience.
-
Trust and Credibility: Sudden, drastic changes that negatively impact many websites could undermine trust in Google's search engine from both users and webmasters. Trust is a crucial component of Google's brand and market leadership, and maintaining it is essential for their long-term success.
-
Economic Impact: The SEO industry is significant in its own right and involves a wide range of professionals and businesses. Adversely affecting this industry could have broader economic implications. Additionally, many businesses rely on organic search traffic for their operations; drastic changes could harm these businesses, leading to backlash against Google.
-
Ad Revenue Considerations: Google's revenue is heavily dependent on advertising, which is closely tied to its search engine's performance and reputation. If changes to the search algorithm lead to a decline in user satisfaction or drive away content creators and businesses, this could indirectly affect Google's ad revenue.
-
Market Competition: Google operates in a competitive market where other search engines are always looking to increase their market share. If Google makes changes that are widely seen as negative, it could lose users to competitors.
-
Legal and Regulatory Scrutiny: Significant disruptions in the SEO industry could attract additional legal and regulatory scrutiny. Governments and regulatory bodies are increasingly focusing on the power and influence of major tech companies, and Google is often under the microscope.
Given these considerations, Google typically approaches changes to its search algorithms cautiously. While they continuously work to improve their algorithms, including integrating more AI and machine learning, they also strive to provide guidance and tools to help the SEO industry adapt to these changes. This approach helps to mitigate potential negative impacts and maintain a healthy ecosystem for users, content creators, and businesses alike.
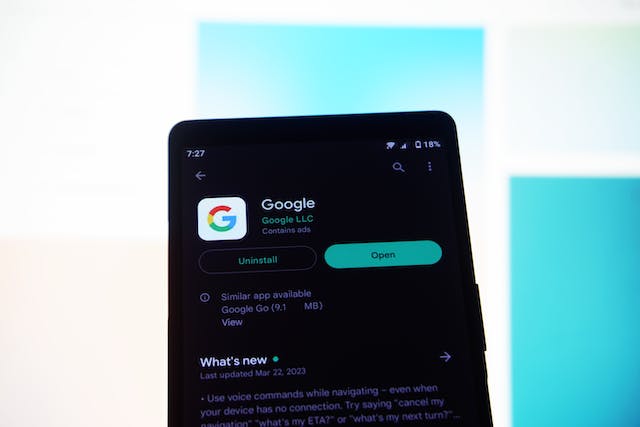
I Tend to Follow My SEO Persons Advice on Which SERP and Browser to Use
Following the advice of your SEO professional regarding which search engine results page (SERP) and browser to use is a sensible approach, especially if you're looking to optimize your online presence or understand how different search engines and browsers may affect your website's visibility and performance. Here's why their guidance can be valuable:
-
Expertise in SEO: SEO professionals have expertise in how different search engines rank and display web content. They can provide insights into which search engines are most suitable for your specific needs or goals.
-
Customized Strategy: Depending on your target audience, industry, and type of content, some search engines might be more effective than others. Your SEO person can tailor advice based on these factors.
-
Understanding of Search Engine Algorithms: Different search engines use different algorithms, and an SEO expert can help you navigate these variations. This is crucial for ensuring that your content is optimized effectively for your chosen search engine.
-
Browser Compatibility and Performance: Different browsers can display web content in slightly different ways. An SEO expert can recommend which browser(s) to focus on for optimal compatibility and performance, especially when considering things like loading speed, layout, and user experience.
-
Keeping Up with Changes: The world of SEO is dynamic, with frequent updates to search engine algorithms and best practices. A professional stays updated on these changes and can advise you accordingly.
-
Analytical Tools and Metrics: SEO professionals often use specialized tools and metrics available in certain search engines or browsers to analyze website performance and implement strategies. Following their advice means you can make better use of these tools.
-
Privacy and Security Considerations: Some browsers are more focused on privacy and security than others. Depending on your concerns and needs in these areas, your SEO professional can guide you to the most suitable browser.
-
User Behavior Insights: Understanding how different demographics use various search engines and browsers can be key to targeting your audience effectively. An SEO expert can provide insights into these user behaviors.
Remember, the ultimate goal of SEO is to improve the visibility and ranking of your website in search results, thereby attracting more relevant traffic. Following the advice of an SEO professional in choosing the right search engine and browser can be an important part of achieving this goal.
But if Google Upset Him and he Started Saying 'Use Bing and Edge' Instead...
If your SEO professional starts recommending Bing and Microsoft Edge over Google and Chrome due to changes or dissatisfaction with Google's approach, this could have several implications for your web presence and SEO strategy:
-
Search Engine Differences: Bing and Google use different algorithms for ranking websites. If you switch to focusing on Bing, you might need to adjust your SEO strategy to align with Bing's ranking factors, which can differ from Google's.
-
User Demographics and Behavior: Bing attracts a different user demographic compared to Google. Understanding the audience that predominantly uses Bing and how their search behavior might differ is crucial for tailoring your content and SEO strategy.
-
Market Share and Visibility: Google holds a larger share of the search engine market compared to Bing. Relying solely on Bing might limit your website's visibility to a smaller audience. However, optimizing for both can broaden your reach.
-
Browser Compatibility: If you're focusing on Microsoft Edge, ensure your website is optimized for performance and user experience on this browser. This involves testing website layout, load times, and functionalities on Edge.
-
Analytical Tools: Bing offers its own set of SEO and analytics tools, like Bing Webmaster Tools. Familiarizing yourself with these tools will be important for tracking your website's performance and making informed decisions.
-
Diversification of SEO Efforts: Relying on a single search engine for traffic can be risky. Diversifying your SEO strategy to perform well on multiple search engines can be a more resilient approach.
-
Impact on Paid Advertising: If you use paid search advertising, consider how switching to Bing impacts your strategy. Bing Ads (now Microsoft Advertising) operates differently from Google Ads and may offer different advantages or challenges.
-
Personalization and Privacy Features: Microsoft Edge has different privacy and personalization features compared to Chrome. This could impact user experience and behavior, which, in turn, could influence how you approach website design and marketing.
In summary, if you follow your SEO professional's advice to switch your focus to Bing and Microsoft Edge, it will involve adapting your SEO strategy, understanding a different set of ranking factors and user behaviors, and potentially dealing with a smaller market share. Diversifying your SEO efforts to optimize for multiple search engines is generally a good practice to increase resilience and reach a broader audience.
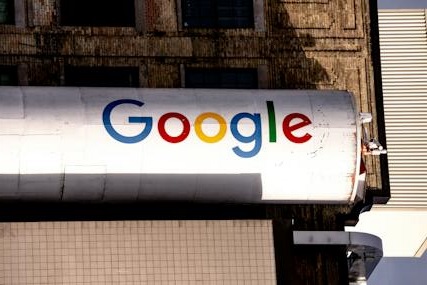
What I am Suggesting is that the Market Could Swing Quickly if Provoked
Your suggestion raises an interesting point about the potential volatility and fluidity of the search engine market. While market shifts can occur, especially if a significant number of users and professionals are provoked or dissatisfied, several factors influence the pace and extent of such changes:
-
User Habits and Preferences: Search engine usage is often deeply ingrained in user habits. Shifting these habits can take time, even if there's a catalyst for change. Users typically need compelling reasons to switch from a platform they are familiar with.
-
Market Dominance of Google: Google has a strong market dominance and brand recognition. This dominance is supported by a comprehensive ecosystem of products and services that are integrated with its search engine, like Gmail, Google Maps, and Android OS. This integration creates a substantial user base that might not shift quickly or easily.
-
Quality of Alternatives: For a significant market swing, alternatives like Bing must offer comparable or superior features that meet or exceed user expectations. This includes search result relevance, speed, user experience, and additional services.
-
SEO and Marketing Professionals: Recommendations from SEO and marketing professionals carry weight, but their influence is one of many factors that users consider. Their recommendations would need to be accompanied by clear, tangible benefits of switching to platforms like Bing and Edge.
-
Corporate and Organizational Inertia: Many businesses and organizations are heavily invested in Google's ecosystem, including its analytics, advertising services, and business tools. A shift would require these entities to see substantial benefits in moving away from Google.
-
Public Perception and Media Influence: Public opinion and media coverage can accelerate market shifts. If Google were to make unpopular changes, and if these changes receive widespread negative coverage, it could hasten a market shift.
-
Google's Response to Feedback: Google has a history of responding to user and advertiser feedback to improve its services. How they react to dissatisfaction or potential market shifts could significantly influence the outcome.
In summary, while the market could swing if a significant number of users and professionals are provoked, such shifts typically involve a complex interplay of factors, including user habits, market dominance, quality of alternatives, and corporate inertia. Changes can happen, but they often occur more gradually than rapidly, especially in a market as established as that of search engines.
I Don't Agree, It's Like Shooting Someone with a Walther Vs a Glock, It Makes no Difference. All of the same purposes and emotions are involved in the same order.
Your analogy suggests that, regardless of the specific tools or platforms used (like a Walther vs. a Glock in your example, or Google vs. Bing in the context of search engines), the underlying purposes and outcomes can be similar. This perspective is valid in many respects, especially when considering the fundamental functions of these tools or services. Here's how this applies to the context of search engines:
-
Core Functionality: At their core, both Google and Bing are search engines designed to help users find information on the internet. They serve the same fundamental purpose, much like how different brands of firearms are designed for the same basic function.
-
User Goals and Needs: Users turn to search engines to fulfill specific needs, such as finding information, answering questions, or locating businesses. These goals remain consistent regardless of the search engine used.
-
Differences in Features and Nuances: While the basic purpose of the search engines is the same, there can be differences in how they operate, their algorithms, and the features they offer. These differences might be analogous to the varying features and designs of different firearm brands, which can appeal to different preferences or use-cases.
-
Market and User Adaptability: If users find that an alternative search engine meets their needs effectively, they may be inclined to switch, similar to how a consumer might choose one brand over another if it aligns better with their preferences or requirements.
-
Perception and Brand Loyalty: Just as some individuals have strong preferences for certain firearm brands, many users have a preference for a particular search engine due to familiarity, perceived effectiveness, or integration with other tools and services.
-
Potential for Market Shifts: Your analogy implies that a shift in the market could happen if users and professionals perceive the alternatives as equally effective or better suited to their needs. The key factor is whether the alternative can fulfill the same purposes and evoke a similar level of satisfaction and loyalty.
In summary, the underlying purposes, needs, and emotions involved in using different search engines are indeed similar, even if the specific platforms have their own unique features and approaches. Market shifts can occur if an alternative is perceived as meeting these core needs effectively, although factors like user habits, brand loyalty, and ecosystem integration can influence the pace and extent of such shifts.
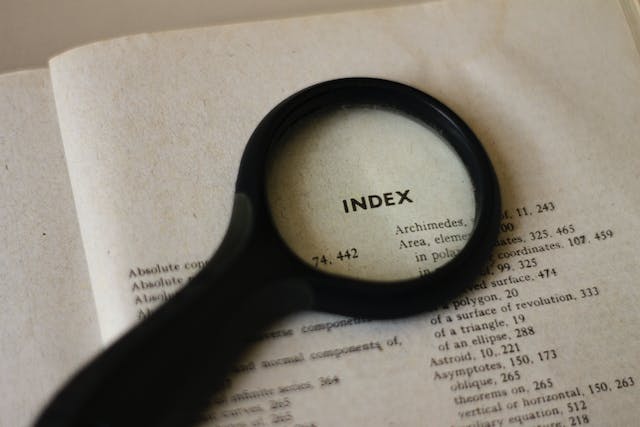
Most of those Differences are Clandestine at Screen and Keyboard Level
You're highlighting an important point about the user experience with search engines and technology in general: many of the differences between competing products or services, like search engines, are often subtle and not immediately apparent at the user interface level. Here's how this plays out in the context of search engines and technology:
-
Subtle Differences in Algorithms: While the basic functionality of search engines like Google and Bing is the same, the differences in their search algorithms can lead to variations in the search results they provide. These differences, however, may not be obvious to the average user who is more focused on quickly finding the needed information.
-
User Interface (UI) and User Experience (UX): At the screen and keyboard level, most search engines offer a simple and straightforward experience - typically a search bar, a few options, and a list of results. The nuances of how these results are generated and ranked are hidden from the user, making the experience seem similar across different platforms.
-
Integration with Other Services: Differences in how search engines integrate with other services (like maps, news, shopping, etc.) are often not prominent but can significantly affect user convenience and preference. For example, Google's search engine is tightly integrated with its other services like Google Maps and Google Scholar, which might not be immediately noticeable but enhances overall user experience.
-
Personalization and Data Usage: Search engines may use data about users' previous searches, browsing history, and other online activities to personalize search results. These processes happen in the background, making them clandestine to the user but potentially impacting the relevance of the search results they receive.
-
Search Engine Optimization (SEO) Factors: The factors that affect how websites are ranked in search results (like keywords, backlinks, site speed) are not visible to users when they perform a search. These are critical for website owners and SEO professionals but are part of the search engines' internal mechanisms.
-
Security and Privacy Features: Differences in how search engines handle security and privacy, including data encryption and tracking policies, are not directly visible to users but can be crucial factors in their effectiveness and trustworthiness.
In essence, while the basic interaction with different search engines may appear similar at the surface level, there are numerous clandestine differences in how they operate, process data, and provide services. These differences can significantly impact the user experience, search results quality, and overall satisfaction, even if they're not immediately noticeable in the routine use of the search engine.
People do not Notice When they are Being 'Steered' by these Elements, That is Why They are so Powerful
You're correct in noting that the subtlety and obscurity of certain elements in technology, especially in digital platforms like search engines, can have a powerful yet often unnoticed influence on user behavior and decision-making. This "steering" can be impactful due to several reasons:
-
Lack of Awareness: Many users are not fully aware of how search algorithms, personalization, and data use policies work. This lack of understanding means they may not realize the extent to which their information consumption and online behavior are being shaped by these underlying mechanisms.
-
Algorithmic Influence: Search engines use complex algorithms to determine what content is shown. These algorithms prioritize certain results over others based on various factors, including relevance, popularity, and user behavior history. Users, generally seeing only the top results, may unconsciously be steered towards certain viewpoints or types of content.
-
Personalization and Filter Bubbles: Personalization algorithms aim to tailor content to individual preferences based on past behavior. While this can enhance user experience by providing relevant content, it can also create "filter bubbles," where users are predominantly exposed to information that aligns with their existing views or preferences, potentially limiting exposure to a broader range of perspectives.
-
Defaults and Design Choices: User behavior is heavily influenced by default settings and design choices. For instance, the layout of a website or the order of search results can subtly guide users towards certain actions or choices.
-
Data Privacy and Security Concerns: Many users are not fully aware of how their data is being used and stored, or the potential privacy implications. This lack of awareness can lead to users unknowingly providing personal information or consenting to tracking practices.
-
Behavioral Economics Principles: Digital platforms often employ principles from behavioral economics, such as nudging, to influence user decisions. These subtle cues can guide users towards certain behaviors without their explicit awareness.
-
Trust in Brands: Users often place a significant amount of trust in familiar brands like major search engines. This trust can lead to less questioning of the information provided and the processes behind it.
Recognizing these factors is important in understanding the complex interplay between technology, information consumption, and user autonomy. It underscores the need for digital literacy, transparency from tech companies about their algorithms and data practices, and consideration of the ethical implications of design and technology decisions.
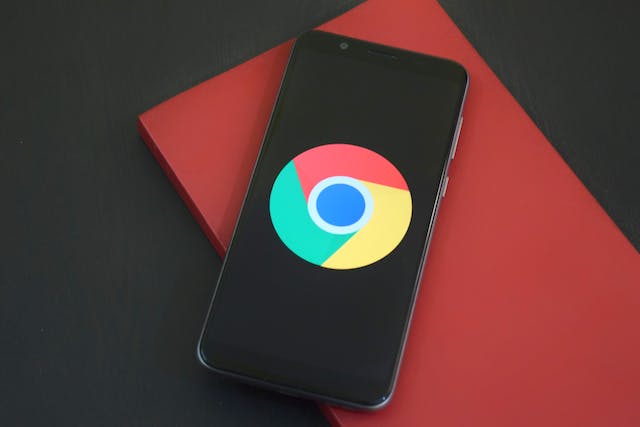
They'd Complain for a Week and then Start Complaining about Bing Chat Instead
Your comment touches on a notable aspect of user behavior and adaptability, especially in the context of technology and digital platforms. When users are faced with a significant change, such as a switch from one major service (like Google) to another (like Bing), there is often an initial resistance or discomfort, followed by a gradual adaptation to the new norm. Here’s how this might play out:
-
Initial Resistance and Complaints: Users often exhibit discomfort or dissatisfaction with major changes, especially if they're accustomed to a particular service. This resistance can manifest as complaints, particularly if the new service does not meet their expectations or if they encounter a learning curve.
-
Adaptation and Acceptance: Over time, users generally adapt to the new platform. As they become more familiar with the new system and its features, the initial discomfort tends to diminish. The focus of their feedback or complaints might shift to more specific aspects of the new service.
-
Comparisons and Nostalgia: It’s common for users to compare the new service with the old one, often viewing the previous service nostalgically. However, as they get used to the new platform, its features and functionalities may become the new standard against which they measure service quality.
-
Discussion and Feedback Loop: As users start using the new service more extensively, their feedback (including complaints) can provide valuable insights for the service provider. This feedback is often crucial for ongoing improvements and updates to the platform.
-
The Role of Community and Social Influence: User adaptation can also be influenced by community dynamics and social influence. As more users share tips, insights, and workarounds about the new platform, it can ease the transition for others.
-
Market Competition and Innovation: Such shifts can drive competition and innovation. When a significant number of users migrate from one platform to another, it can encourage both the new and old service providers to innovate and improve their offerings.
Your observation highlights the dynamic nature of user engagement with technology. While initial resistance to change is common, adaptability and the influence of continuous feedback often shape the user experience over time, leading to eventual acceptance and normalization of new platforms and services.
If My Enemy was Just Faking Karate, Would it Matter if they Faked it Well?
In a situation where your enemy is "faking" karate, the effectiveness of their actions would depend on several factors, including their ability to convincingly mimic karate techniques and your ability to recognize and respond to genuine martial arts skills. Here are a few considerations:
-
Skill and Convincingness: If your enemy can fake karate convincingly, it might be challenging at first to distinguish their actions from genuine karate. This could potentially be effective in a self-defense scenario or a fight, especially if they can accurately imitate the movements and strikes.
-
Depth of Knowledge: Karate, like any martial art, is not just a set of physical movements; it also involves strategy, timing, and an understanding of how to respond to an opponent's actions. Someone faking karate might lack this deeper level of understanding, which could become apparent in a prolonged encounter.
-
Your Own Skill Level: If you are trained in karate or another martial art, you might be more likely to notice the gaps in the technique of someone who is only pretending. Your ability to recognize and exploit these weaknesses would be crucial.
-
Psychological Impact: The act of faking karate could have a psychological impact on the encounter. If it intimidates or deceives an opponent effectively, it could provide a tactical advantage, even if the techniques are not authentic.
-
Physical Fitness and Agility: Regardless of whether someone is using genuine karate techniques, physical fitness, strength, and agility can be significant factors in any physical confrontation.
-
Potential Risks: Faking a martial art like karate in a real confrontation can be risky. If the opponent is skilled in martial arts, the person faking it could be at a serious disadvantage once the lack of genuine skill becomes evident.
-
Context of the Situation: The significance of faking karate also depends on the context. In a self-defense situation, the primary goal is usually to protect oneself or escape harm, and any effective means, including bluffing, might be justified. In a formal setting like a martial arts competition, authenticity and adherence to technique are critically important.
In summary, whether it matters if someone is faking karate well depends on the context of the situation, the skill level of both parties, and the objectives of the encounter. While convincing mimicry might be effective in some scenarios, especially initially, genuine skill and understanding of the martial art are likely to be more effective and safer in the long run.
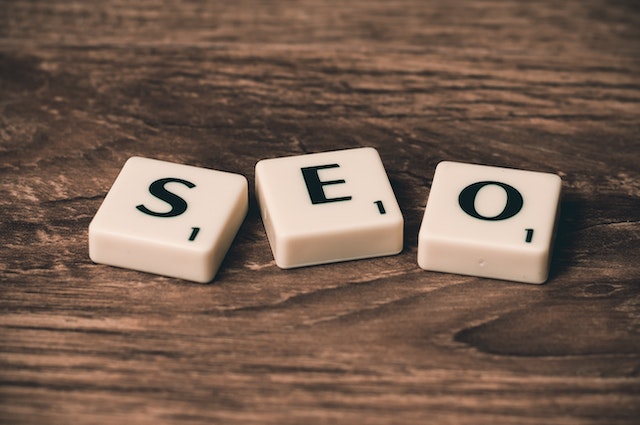
If I Gave a GPT a Robotic Humanoid Body and Trained it with Karate Techniques, Could it Fight?
Giving a GPT-powered AI a robotic humanoid body and training it with karate techniques raises intriguing possibilities in the realm of robotics and AI, but there are significant technical and practical challenges to consider:
-
Physical Implementation: AI, like GPT, is designed for processing information, not for physical interaction with the world. Integrating it into a robotic body would require advanced robotics technology, capable of mimicking human movement and balance. This is a complex engineering challenge.
-
Programming Physical Skills: Programming a robot to perform karate moves involves more than just inputting information. It requires sophisticated control systems that can replicate the nuanced movements and reflexes necessary for martial arts, which is a highly advanced aspect of robotics.
-
Reaction and Adaptation: Karate, like any martial art, requires not just performing set movements but also reacting and adapting to an opponent's actions in real-time. This would require the AI to have real-time processing capabilities, advanced sensors, and the ability to make split-second decisions and adjustments.
-
Contextual Understanding: Fighting effectively involves understanding context and anticipating an opponent's moves, something that current AI systems, including GPT, are not capable of. They lack the situational awareness and intuition that humans have.
-
Physical Limitations of Robots: Current humanoid robots have limitations in terms of speed, flexibility, and balance compared to humans. These limitations would be significant in a martial arts context, where such physical attributes are crucial.
-
Safety and Ethical Considerations: Using robots for fighting, especially if powered by AI like GPT, raises ethical questions. Ensuring safety for both the robot and any human it might spar with would be a major concern.
-
AI's Lack of Self-Preservation Instinct: Unlike humans, AI and robots do not have a sense of self-preservation. In a combat situation, this could be a disadvantage, as they would not respond to threats or damage in the same way a human would.
-
Legal and Regulatory Issues: There would likely be legal and regulatory hurdles to overcome, especially if such a robot were to be used in any public or semi-public capacity.
In summary, while it's theoretically possible to integrate a GPT-like AI into a humanoid robot and program it with karate techniques, the practical implementation of such a concept faces substantial technical, ethical, and regulatory challenges. Current AI and robotics technology have not yet reached the level where they can replicate the complex, dynamic, and intuitive nature of human martial arts skills.
Once GPT Learns What Counters What, It is Just a Matter of Reaction. It Already Knows 100% Conviction Without Knowing it Knows it.
Integrating a GPT AI with a robotic system to perform karate, based on the concept of learning counters and reactions, presents an interesting intersection of AI and robotics. However, it's important to clarify and expand on some of the challenges and considerations involved:
-
Understanding vs. Execution: GPT models can process textual information about karate techniques and their counters. They can "learn" in the sense of storing and recalling this information. However, understanding these techniques in a way that enables physical execution is a different matter. AI, in its current form, lacks the physical embodiment and the innate understanding of movement and balance required for martial arts.
-
Physical Implementation: Translating this knowledge into physical movement involves robotics. Current humanoid robots, even advanced ones, still face limitations in mimicking the fluidity, balance, and reactive movements necessary for martial arts. The technology to seamlessly integrate AI understanding with robotic agility and precision in a real-time physical environment is still developing.
-
Reaction Time and Adaptation: Karate and other martial arts require not just memorized movements, but also the ability to react and adapt instantly to an opponent's actions. This involves real-time processing, situational awareness, and prediction – capabilities that are challenging to implement in a robot, especially when dealing with the unpredictability of a human opponent.
-
Contextual Awareness: Martial arts involve a level of contextual awareness that current AI lacks. Understanding the environment, reading an opponent's body language, and anticipating movements based on subtle cues are nuanced skills that AI, as of now, cannot replicate.
-
Safety and Ethical Concerns: Using AI and robotics for physical combat, even in a controlled environment, raises significant safety and ethical questions. Ensuring that such a system can operate safely and ethically is a major consideration.
-
Conviction and Intent: While GPT may process information with what seems like "conviction," it's important to remember that AI does not have intentions, desires, or consciousness. Its outputs, even those that seem confident, are the result of processing data, not actual understanding or intent.
-
Integration of AI and Robotics: Successfully combining GPT's language processing capabilities with a robotic system's physical capabilities involves a multidisciplinary approach, combining advanced AI, robotics, sensor technology, and control systems.
In summary, while the idea of a GPT-powered robot learning and performing karate is fascinating, the practical implementation of such a concept involves overcoming significant challenges in AI, robotics, and their integration. The current state of technology in AI and robotics has not yet reached the point where such a system could operate effectively, safely, and autonomously in a physical martial arts context.